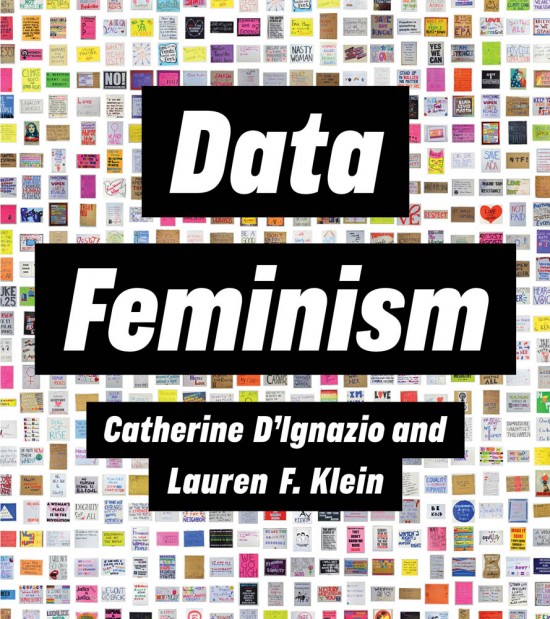
Today, data science is a form of power. It has been used to expose injustice, improve health outcomes, and topple governments.
But it has also been used to discriminate, police and surveil.
This potential for good, on the one hand, and harm, on the other, makes it essential to ask: Data science by whom? Data science for whom? Data science with whose interests in mind?
The narratives around big data and data science are overwhelmingly white, male and techno-heroic.
In our new book, Data Feminism, we present a new way of thinking about data science and data ethics—one informed by intersectional feminist thought.
Illustrating data feminism in action, we show how challenges to the male/female binary can help challenge other hierarchical—and empirically wrong—classification systems.
For example, we explain how an understanding of emotion can expand our ideas about effective data visualization—and how the concept of invisible labor can expose the significant human efforts required by our automated systems.
And we show why the data never, ever “speak for themselves.”
Data Feminism offers strategies for data scientists seeking to learn how feminism can help them work toward justice, and for feminists who want to focus their efforts on the growing field of data science. But Data Feminism is about much more than gender. It is about power, about who has it and who doesn’t, and about how those differentials of power can be challenged and changed.
Below is an excerpt from Chapter 1 of Data Feminism which outlines the unknowingly racist, sexist underpinnings of data systems many of us have come to grow and love—or, at least, tolerate.
Let’s take a minute to imagine what life is like for someone who epitomizes the dominant group in data science: a straight, white, cisgender man with formal technical credentials who lives in the United States. When he looks for a home or applies for a credit card, people are eager for his business. People smile when he holds his girlfriend’s hand in public. His body doesn’t change due to child birth or breastfeeding, so he does not need to think about workplace accommodations. He presents his social security number in jobs as a formality, but it never hinders his application from being processed or brings him unwanted attention. The ease with which he traverses the world is invisible to him because it has been designed for people just like him. He does not think about how life might be different for everyone else. In fact, it is difficult for him to imagine that at all.
This is the privilege hazard: the phenomenon that makes those who occupy the most privileged positions among us—those with good educations, respected credentials, and professional accolades—so poorly equipped to recognize instances of oppression in the world. They lack what Anita Gurumurthy, executive director of IT for Change, has called “the empiricism of lived experience.” And this lack of lived experience—this evidence of how things truly are—profoundly limits their ability to foresee and prevent harm, to identify existing problems in the world, and to imagine possible solutions.
The privilege hazard occurs at the level of the individual—in the interpersonal domain of the matrix of domination—but it is much more harmful in aggregate because it reaches the hegemonic, disciplinary and structural domains as well. So it matters deeply that data science and artificial intelligence are dominated by elite white men because it means there is a collective privilege hazard so great that it would be a profound surprise if they could actually identify instances of bias prior to unleashing them onto the world. Social scientist Kate Crawford has advanced the idea that the biggest threat from artificial intelligence systems is not that they will become smarter than humans, but rather that they will hard-code sexism, racism, and discrimination into the digital infrastructure of our societies.
What’s more, the same cis het white men responsible for designing those systems lack the ability to detect harms and biases in their systems once they’ve been released into the world. In the case of the “three teenagers” Google searches, for example, it was a young Black teenager that pointed out the problem and a Black scholar who wrote about the problem. The burden consistently falls upon those more intimately familiar with the privilege hazard—in data science as in life—to call out the creators of those systems for their limitations.
For example, Joy Buolamwini, a Ghanaian-American graduate student at MIT, was working on a class project using facial-analysis software. But there was a problem— the software couldn’t “see” Buolamwini’s dark-skinned face (where “seeing” means that it detected a face in the image, like when a phone camera draws a square around a person’s face in the frame). It had no problem seeing her lighter-skinned collaborators. She tried drawing a face on her hand and putting it in front of the camera; it detected that. Finally, Buolamwini put on a white mask, essentially going in “whiteface” (figure 1.3). The system detected the mask’s facial features perfectly.
Digging deeper into the code and benchmarking data behind these systems, Buolamwini discovered that the dataset on which many of facial-recognition algorithms are tested contains 78 percent male faces and 84 percent white faces. When she did an intersectional breakdown of another test dataset—looking at gender and skin type together—only 4 percent of the faces in that dataset were female and dark-skinned. In their evaluation of three commercial systems, Buolamwini and computer scientist Timnit Gebru showed that darker-skinned women were up to forty-four times more likely to be misclassified than lighter-skinned males. It’s no wonder that the software failed to detect Buolamwini’s face: both the training data and the benchmarking data relegate women of color to a tiny fraction of the overall dataset.
This is the privilege hazard in action—that no coder, tester, or user of the software had previously identified such a problem or even thought to look.
You may also like: