We have a long way to go before women share equally in the creation of the technologies that will change the future.
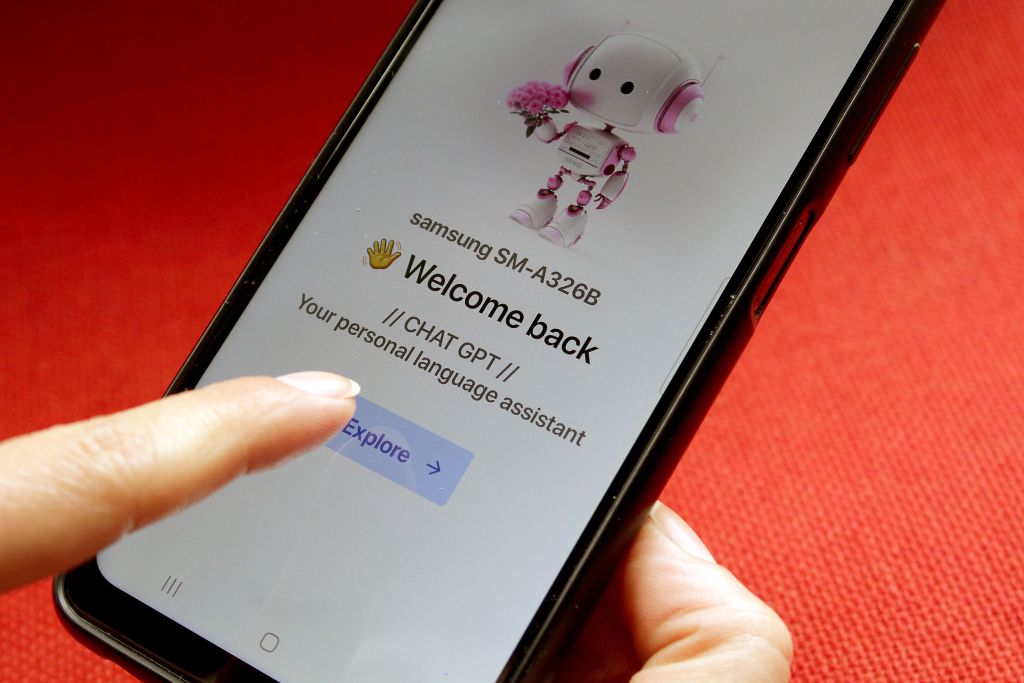
New advancements in data science often spark dire predictions about how powerful new technologies will transform the world. Yet, as writer Stephen Shankland reminds us, technologies like Open AI’s new Chat GPT (short for chat-based Generative Pretrained Transformer) are created by humans.
Chat GPT is a chatbot that is “trained with human assistance to deliver more useful, better dialog.” The people assisting that training—those who create the models and assemble the data used to train chatbots—make a difference in the technologies that will go on to shape our lives.
Computer scientist Joy Buolamwini, an early critic of racial bias in facial recognition software, said technology should “be more attuned to the people who use it and the people it’s used on.” But as long as the field of data science remains predominantly male and white, it will be difficult to have inclusive advancements in artificial intelligence. A new white paper by Women in Data Science (WiDS) at Stanford University, “Identifying and Removing Barriers for Women to Pursue Graduate Degrees in Data Science and AI,” shows that we have a long way to go before women share equally in the creation of the technologies that will change the future.
Why, despite decades of pipeline programs created to address the lack of gender diversity in computer and information sciences, are only 17 percent of those enrolled in Ph.D. programs women? The white paper contends that the proverbial pipeline is not so much leaky as it is blocked by barriers that prevent women from pursuing graduate studies in data science and AI (the authors focus on graduate programs since most successful data scientists today have advanced degrees).
Some of these barriers are frustratingly familiar. Chief among these is a lack of awareness among undergraduates about academic programs that can lead to careers in data science, a related lack of awareness of the value of a graduate degree in data science (the median entry-level annual salary is $95,000), and the lack of awareness of the impact women can have on society as data scientists.
Because there are so few women in the field, undergraduate women rarely see role models who can help them imagine careers in data science and graduate students cannot work with women mentors who can support them as they complete their degrees.
A lack of family, peer and community support also presents barriers for women. Women who are expected to provide financial support to their families, are low-income, or are first-generation students may not receive support from communities that are also unlikely to be aware of the value of a graduate degree. First-generation students in particular do not have access to the tools they will need to navigate academic programs in which they are a minority.
A male-dominated data science culture paired with a lack of female role models can also create an environment conducive to self-doubt and impostor syndrome for young, female data scientists. Self-efficacy is the belief that you can accomplish desired goals and objectives, even when presented with adversity. As the WiDS white paper shows, high-achieving female students report lower self-efficacy in male-dominated STEM fields relative to both their male peers and average-performing male students.
Believing that they are unfit and unwelcome in an academic program creates feelings of inadequacy among female data scientists, with negative impacts on their confidence and self-esteem. Capable and talented women dealing with low self-efficacy and impostor syndrome often leave data science and related fields at the undergraduate level, resulting in under-enrollment in graduate programs. They also leave programs at higher rates before completing a degree. Internal barriers can be just as harmful as external barriers, but they are perhaps easier to overcome with support and inclusion from mentors and peers.
When data scientists do not bring diverse perspectives to their work, the science and technology they produce suffers.
Some solutions have been proposed and implemented at the undergraduate level to bridge the gender gap in advanced degree enrollment in data science. The white paper suggests that a critical threshold of at least 30 percent of those working in computer and information science should identify as women to realistically enforce equity and inclusion initiatives within the field.
In order to reach this threshold within the next decade, WiDS has proposed the creation of the Women in Data Science Academy, a cross-university program that intends to both increase awareness of the avenues available to pursue advanced degrees in data science, as well as encourage women to forge a direct path from their undergraduate to graduate studies.
The WiDS Academy plans to pair technical-skills-based initiatives to strengthen coding literacy with career-oriented programs like graduate school recruitment initiatives and career talks with women working and conducting research in the data science field. These initiatives are designed to increase awareness and self-efficacy and try to decrease feelings of impostor syndrome among undergraduate students. Although this academy alone will not be able to address the barriers that women face in this field, by presenting a path for talented and qualified women to pursue advanced data science degrees and a model for other institutions to adopt, it might yet be possible to reach the critical threshold of 30 percent within the decade.
As the white paper shows, the representation of women in data science worldwide is “dismal.” When data scientists do not bring diverse perspectives to their work, the science and technology they produce suffers. Not only are women being prevented from entering a dynamic and high-paying field, but the lack of diversity also results in a loss of creativity and innovation in data science. Lack of inclusion hurts women and the subsequent lack of diversity hurts science. The white paper offers us solutions.
Up next:
U.S. democracy is at a dangerous inflection point—from the demise of abortion rights, to a lack of pay equity and parental leave, to skyrocketing maternal mortality, and attacks on trans health. Left unchecked, these crises will lead to wider gaps in political participation and representation. For 50 years, Ms. has been forging feminist journalism—reporting, rebelling and truth-telling from the front-lines, championing the Equal Rights Amendment, and centering the stories of those most impacted. With all that’s at stake for equality, we are redoubling our commitment for the next 50 years. In turn, we need your help, Support Ms. today with a donation—any amount that is meaningful to you. For as little as $5 each month, you’ll receive the print magazine along with our e-newsletters, action alerts, and invitations to Ms. Studios events and podcasts. We are grateful for your loyalty and ferocity.